A Hyperspectral-Based System for Identification of Common Bean Genotypes Resistant to Foliar Diseases in Tanzania.
- March 29, 2024
- Godfrey Naman
- 0
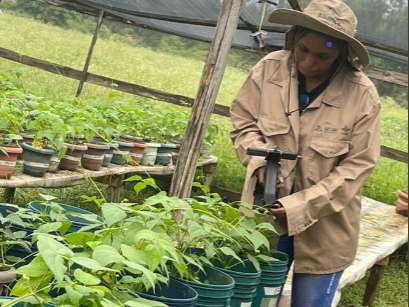
Research Problem
Common bean is one of many legumes (family Fabaceae) widely cultivated for their edible seeds, seedpods, and leaves. Despite its benefits and life dependency especially in sub-Saharan Africa, due to climatic change, high population expansion, harsh weather conditions, and other disasters including diseases are causing considerable loss, where only foliar diseases are causing 20 to 80% of common bean production loss every year.
To overcome these challenges, bean-breeding programs were established prioritizing the development of new seed varieties to ensure high production and yield stability under adverse environmental conditions. High-yielding and resilient seed varieties are known from their observable traits which are measured through the process of phenotyping. However, development of improved common bean seeds is still a problem where it takes up to ten years to develop a new bean variety.
The major challenge Tanzanian bean breeders are facing is manual phenotyping, which is a tiring slow field process with manual paper writing as data collection tool and has many data errors, data loss, and data biases as results depend on the eyes of the viewer.
To increase and optimize the utilization of technology in agriculture for quick better seeds identification, this study introduces the use of artificial intelligence with hyperspectral data to help breeders in the classification of disease damage extent and timely plant health monitoring that will help them in the early identification of bean varieties resistant to foliar diseases and decision making using automated phenotypic data analysis reducing time used to spend on field and on manual data analysis.
Findings
This study developed a unique hyperspectral data-based system composed of a Mobile Application for data collection, classification Algorithm for diseases extent and a Web Application for identifying bean genotypes resistant to foliar diseases and plant health monitoring. Using the Random Forest classifier this study proved the genotype classification in three main breeding categories; Resistant, Medium, and Susceptible. The experiment was conducted in four Regions of Tanzania known for bean breeding (Arusha, Mbeya, Kagera and Manyara). Three classifiers “Random Forest, XgBoost, and four layers Neuro Network algorithms” were trained and tested with results of 0.96, 0.95, and 0.92 respectively. The model was deployed on the cloud server where it is linked to the database and a web application for easy classification and data analysis. Applying different vegetation indexes including the Chlorophyll Index, Photochemical Reflectance Index, Water Band Index, Modified Chlorophyll Absorption in Reflectance Index, Nitrogen Reflectance Index, Structure Insensitive Pigment Index, and Simple Ratio proved to be used for plant health insight before disease symptoms are seen. This saves breeders time, reduces errors, and helps them with digital phenotypic data, faster analysis, and easy storage for future references.
Impact
The findings of this study, as well as the development of the classification of foliar diseases damage to bean plants and categorizing different varieties of common bean genotypes, will help the breeders and the country in general so that farmers can be given trusted seeds in shorter time and regional-based, restoring hope to common bean growers who are depressed due to the expenses and damages brought on by production-related diseases, leading to climate change adaptation and meeting the consumption demand. Therefore, the solution will lessen the workload of the nation’s few researchers and skilled breeders because it is automated and results will not require human intervention. It will also foster the Alliance of Bioversity and CIAT ARTEMIS project goal of Imaging Technology for a food secure future.
Research Credits
I express my tremendous appreciation to my amiable, ever-supportive, and humble academic supervisors, Prof. Shubi Kaijage, Dr. Judith Leo, and Ms Hope Mbelwa, for their invaluable contributions and instructions in developing this project.
I am highly thankful to my industrial supervisors Dr. David Guerena and Dr. Teshale Mamo, who took their time to introduce and guide me into Agriculture Sector, and helped me during the research from the beginning to the completion of this work.
Special thanks are extended to the Nelson Mandela African Institution of Science of Technology (NM-AIST), the Center of Excellence for Information Communication and Technology in East Africa (CENIT@EA), and Deutscher Akademischer Austauschdienst (DAAD) Deutsche Gesellschaft für Internationale Zusammenarbeit GmbH (GIZ)’s scholarship program.
I express my big appreciation to The Alliance of Bioversity and International Center for Tropical Agriculture (CIAT), ARTEMIS Project for funding this research and providing me with a facility and a conducive environment that helped me to complete this project successfully.